- Published by:
- Department of Treasury and Finance
- Date:
- 1 Mar 2024
Madeleine Tan1 2
1. Department of Treasury and Finance
2. The author would like to thank Xinyi Tang, Jeffrey H Wong and Rebecca Valenzuela for their assistance. The paper has greatly benefitted from comments from Prof. Alicia Rambaldi and Andrew O’Keefe.
Author contact details: veb@dtf.vic.gov.au.
Disclaimer: The views expressed are those of the author and do not necessarily reflect the views of the Victorian Department of Treasury and Finance (DTF).
Suggested Citation: Madeleine Tan (2024), Understanding the impact of settlement lags and data revisions on Victoria’s property market projections. Victoria’s Economic Bulletin, March 2024, vol 8, no 1. DTF.
Abstract
Developing forecasts and making predictions can prove challenging in an environment of constantly changing information. An essential component of making good decisions is understanding what is not yet known. Property market data is a good example of this. Transactions data, specifically, can present issues because data on transacted properties is available only at the point of settlement rather than at contract signing. This delay, also known as the settlement lag, can range from several weeks to months. This means that only a partial view of the market is available to forecasters and modellers when decisions need to be made and can differ from the choice that would have been selected with a full view of the market, only available at a much later date.
In this paper, we systematically examine property data to understand the impact of settled sales data revisions on property market estimates in Victoria. Findings from this study will either affirm our current approach of using real-time data for forecasting or serve as an impetus for rethinking our approach in forecasting in the property space.
The analysis relies on a real-time data approach to assess the differences in forecasts across data releases. First, it looks at identifying segments of the market (e.g. by location, property type and contract price) that make up a larger share of earlier releases of the data. As the first few data releases often significantly influence decisions, these segments hold more sway in the decision‑making process. Next, the impacts of each additional data release are explored. This is done by quantifying the changes observed in a hedonic housing price index with each data release.
We find that higher value properties make up a bigger share of the early data received on transacted properties, while the share of properties by location and type appeared to be stable over vintages. With approximately 60 per cent of transactions received in the first data release on average, this appears to hold enough information to indicate that results from a hedonic price index do not change significantly. The DTF hedonic price index (HPI) was revised up by about 0.6 percentage points over the data releases over a 12-month period.
As the data is currently quite limited in span of time, it is worthwhile revisiting the analysis as more data releases become available under varying property market conditions, to see whether the results change.
1. Introduction
Developing forecasts and making predictions for better decision-making can prove challenging in an environment of constantly changing information.
Forecasts based on information available at one point in time will only support the same decisions or actions in another if the underlying information has remained virtually unchanged. However, available information can be prone to changes. By this logic, the best forecasts and consequent decisions are those that can account for what is not yet known.
Property market data, specifically settled sales data, are often the basis of analysis that policy and decision-making rely on. However, information on property market transactions is only received at the point of settlement rather than at contract signing. This is also known as the settlement lag. Except for off-the-plan transactions that take between two to three years to settle, the period between contract signing and settlement could be a delay of weeks if not months. The first release of the settled sales property market data for a given contract period is therefore only a partial view of all the contracts that have been signed in the same period. This first release also only reflects the transactions that have had the shortest settlement period. From our calculations of the CoreLogic settled sales data, on average only about 60 per cent of transactions for a given contract period are received in the first release.
To our knowledge, there have not been many studies that have investigated the impact of settlement lag. It is unclear to what extent settlement lag affects analyses between initial first data release and the final data release, when all transaction data for a given contract period has been received. It is also not known which segments of the property market dominate the early data releases and therefore influence inferences from early analyses as well as influence decision-making to a greater extent.
This paper complements the work of Anenberg and Laufer (2017), which constructs a house price index based on house values at contract date, when the sale price is determined, rather than on closing or settlement data when the property is transferred. The focus of this paper is to interrogate various data vintages to understand the impact of settlement lags and data revisions on house price forecasts. It first attempts to identify segments of the market that make up a larger share of earlier data releases.
The location of properties (i.e. inner, metropolitan, outer rings of Melbourne or regional Victoria) is first explored to examine whether settled properties in a certain region make up a larger share of the early data releases. This is then followed by analysis on whether the type of property (i.e. houses or units) settle earlier. In the third exploration, the price distribution of each data release is considered by analysing the median contract price for each data release, to determine if early data releases include more properties that have a higher or lower contract price.
Finally, the impact of each data release is explored through the changes observed in a hedonic price index. This is done because price indices are often used in policy and decision-making to quantify and capture price changes and turning points in the property market. As the timing and information content of each data release matters, the analysis uses a real-time data framework. Data releases are also known as vintages and the reference period refers to the period during which contract dates fall.
The next section provides a short discussion about property data and the settlement lag. Section 2 provides a succinct overview of the economic literature and discusses common issues in the property market data space. Section 3 describes the data and approach taken, while Section 4 describes the results from the data investigations. Section 5 then provides some concluding remarks with some avenues of further research.
2. Settlement lag, real time data framework and some previous works
There are often three issues that researchers face when working with property market data.
They are compositional changes, quality improvements and timeliness of the data available (RBA, 2004). These issues are not unique to Australia as similar challenges can be found in most advanced economies in the world as well. We take each one in turn below.
2.1 Compositional changes
At any given point in time, only a certain segment of the property market is transacted. Thus, the type of properties being transacted at any given time could drive price changes if compositional changes between periods are not considered. For example, if there are more 4-bedroom houses sold in the current period but there were more 1-bedroom units sold in the period before, the average or median price could see a significant increase even if no other price drivers have changed.
2.2 Quality improvements
Over time, properties in the national dwelling stock are constantly being improved to meet housing preferences. Improvements could include upgrades in the form of additional bedrooms, bathrooms or additional features that add to the value of the property itself. Thus, these improvements will be captured over time through a property price measure.
2.3 Timeliness
Unlike financial market data, property market transactions data are often only available at the point of settlement rather than contract signing. The Reserve Bank of Australia states that this is likely due to the decentralised nature of the market and the data relied on is often government administrative data, which is available only after settlement (RBA, 2004). Therefore, the most recent data will capture property market activity with a delay. This is also known as the settlement lag.
The settlement lag is directly related to the length of time between the contract signing and the point at which monies are transferred and keys are handed over, i.e. the settlement period. The length of a settlement period can be determined by a variety of factors which are unique to buyers’ and sellers’ circumstances. According to Asabere & Huffman (1993), such factors can include potentially having a longer than normal search for financing and delays on the sale of an existing house. They note that a longer settlement period poses an opportunity cost for the seller but may be beneficial if the seller is an owner-occupier who expects that it will take a while for them to find their next property to live in. The length of the settlement will likely depend on the complexity of the transaction to allow for the completion of all legal and financial paperwork and due diligence prior to settlement, as there are often severe financial penalties for not complying within the stated timeframe.
According to Canstar (2022), the average settlement period is between 30 and 90 days in Victoria and most other states and territories across Australia. This is consistent with the findings of a joint research project conducted by PEXA and Domain which found that the lag between buyer demand (defined by database searches and settlement activity) was approximately eight weeks in NSW and 11 weeks in Victoria (PEXA Insights, 2021).1 But while a typical transaction can settle anywhere between 30 and 90 days, the settlement period can have a long tail as there is a segment of these transactions that is highly complex and will require more time for settlement to occur. Additionally, off‑the-plan sales will also have a longer settlement period relative to sales of existing dwellings. The settlement period for off-the-plan apartments can be up to two to three years according to Shoory (2016).
Given that policy and financial decisions are based on the information set available at a particular point in time, revisions to the information set that significantly change inferences or alter desired policy outcomes could be problematic. To our knowledge, other than PropTrack (2022) there have not been any studies that have attempted to determine whether observing only partial snapshots in early data releases (or vintages) significantly change inferences based on final vintage data (i.e. when all transactions have been received). To conduct such a study, analysis will need to rely on a framework that allows for both time and the information content to be studied.
A burgeoning area in literature focuses specifically on real‑time economic and fiscal data to understand the impact of data revisions on forecasting, monetary policy and fiscal policy. Croushore (2011) provides a review of this literature on the topic of data revisions on monetary policy, specifically. It is with this framework in mind that the analysis is undertaken in our report.
In our investigations of the data vintages, we segment the property market in three ways. Firstly, through a location lens which is in line with the Alonso-Muth-Mills (Alonso, 1964; Muth, 1969; Mills, 1967; Wheaton, 1974) model. This differentiates properties according to their distance to the central business district (CBD) of a city. Secondly, by differentiating types of property, between houses (detached) versus units (non-detached). And thirdly, by the contract price itself. We look at the median contract price for each vintage to determine whether higher or lower value property sales for a given contract period make up a larger share of early vintages.
There are equally probable reasons for why each of these segments may or may not have a shorter settlement period and may be captured in the earlier vintages. Therefore, we go into this investigation without any priors.
Footnotes
[1] This analysis is however only limited to transactions that were originally posted on the Domain website that settled through the PEXA system.
3. Data and methodology
This study uses CoreLogic’s property settled sales transactions data, received on a monthly basis between October 2019 and April 2023.
Relying on real-time analysis terminology, each data release is referred to as a ‘vintage’. For example, the first vintage for settled sales contracted in 2019 Q3 will be the October 2019 data release. This is the first monthly data release of settled sales data for the full quarter of 2019 Q3 (up to and including September 2019) that is available. In this example, 2019 Q3 is the ‘reference quarter.’
Based on separate pieces of analysis, almost all transactions are expected to be settled by the twelfth month. A check of the number of transactions at the 12-month mark shows that it is similar to the number of transactions at the 2-year mark. This suggests that the off-the-plan sales that are anticipated to settle only after two or three years (Shoory, 2016) do not make up a significant portion of the market. Therefore, we assume that the final vintage is released at least 12 months after the first vintage is released. For example, the final vintage for the 2019 Q3 reference quarter will be the October 2020 vintage.
To standardise the real-time data for analysis, vintages are described in terms of the number of months ahead relative to the reference quarter plus one month, e.g. the first vintage for the 2019 Q3 reference quarter is the October 2019 data release, which is 0 months ahead. Table 1 summarises the data vintages and number of months ahead in relation to each reference quarter.
In Victoria, there are approximately 10,000 residential transactions on average that settle each month. Figure 1 summarises the volume of transactions that are received over time for each reference quarter while Table 2 displays the share of transactions that are observed relative to final vintage, as an average over the reference quarters. For a given reference quarter, approximately 60 per cent of total settled property transactions present in the final vintage is observed in the first vintage, on average. By the end of the third month, most of the transactions had been received, which is consistent with the average settlement period from Canstar (2022).
Table 1: Data vintages and months ahead by reference quarter
0m | 1m | 2m | 3m | 4m | 5m | 6m | 7m | 8m | 9m | 10m | 11m | 12m | |
2019 Q3 | Oct 19 | Nov 19 | Dec 19 | Jan 20 | Feb 20 | Mar 20 | Apr 20 | May 20 | Jun 20 | Jul 20 | Aug 20 | Sep 20 | Oct 20 |
2019 Q4 | Jan 20 | Feb 20 | Mar 20 | Apr 20 | May 20 | Jun 20 | Jul 20 | Aug 20 | Sep 20 | Oct 20 | Nov 20 | Dec 20 | Jan 21 |
2020 Q1 | Apr 20 | May 20 | Jun 20 | Jul 20 | Aug 20 | Sep 20 | Oct 20 | Nov 20 | Dec 20 | Jan 21 | Feb 21 | Mar 21 | Apr 21 |
2020 Q2 | Jul 20 | Aug 20 | Sep 20 | Oct 20 | Nov 20 | Dec 20 | Jan 21 | Feb 21 | Mar 21 | Apr 21 | May 21 | Jun 21 | Jul 21 |
2020 Q3 | Oct 20 | Nov 20 | Dec 20 | Jan 21 | Feb 21 | Mar 21 | Apr 21 | May 21 | Jun 21 | Jul 21 | Aug 21 | Sep 21 | Oct 21 |
2020 Q4 | Jan 21 | Feb 21 | Mar 21 | Apr 21 | May 21 | Jun 21 | Jul 21 | Aug 21 | Sep 21 | Oct 21 | Nov 21 | Dec 21 | Jan 22 |
2021 Q1 | Apr 21 | May 21 | Jun 21 | Jul 21 | Aug 21 | Sep 21 | Oct 21 | Nov 21 | Dec 21 | Jan 22 | Feb 22 | Mar 22 | Apr 22 |
2021 Q2 | Jul 21 | Aug 21 | Sep 21 | Oct 21 | Nov 21 | Dec 21 | Jan 22 | Feb 22 | Mar 22 | Apr 22 | May 22 | Jun 22 | Jul 22 |
2021 Q3 | Oct 21 | Nov 21 | Dec 21 | Jan 22 | Feb 22 | Mar 22 | Apr 22 | May 22 | Jun 22 | Jul 22 | Aug 22 | Sep 22 | Oct 22 |
2021 Q4 | Jan 22 | Feb 22 | Mar 22 | Apr 22 | May 22 | Jun 22 | Jul 22 | Aug 22 | Sep 22 | Oct 22 | Nov 22 | Dec 22 | Jan 23 |
2022 Q1 | Apr 22 | May 22 | Jun 22 | Jul 22 | Aug 22 | Sep 22 | Oct 22 | Nov 22 | Dec 22 | Jan 23 | Feb 23 | Mar 23 | Apr 23 |
2022 Q2 | Jul 22 | Aug 22 | Sep 22 | Oct 22 | Nov 22 | Dec 22 | Jan 23 | Feb 23 | Mar 23 | Apr 23 | |||
2022 Q3 | Oct 22 | Nov 22 | Dec 22 | Jan 23 | Feb 23 | Mar 23 | Apr 23 | ||||||
2022 Q4 | Jan 23 | Feb 23 | Mar 23 | Apr 23 | |||||||||
2023 Q1 | Apr 23 |
Figure 1: Volume of settled sales transactions across vintages
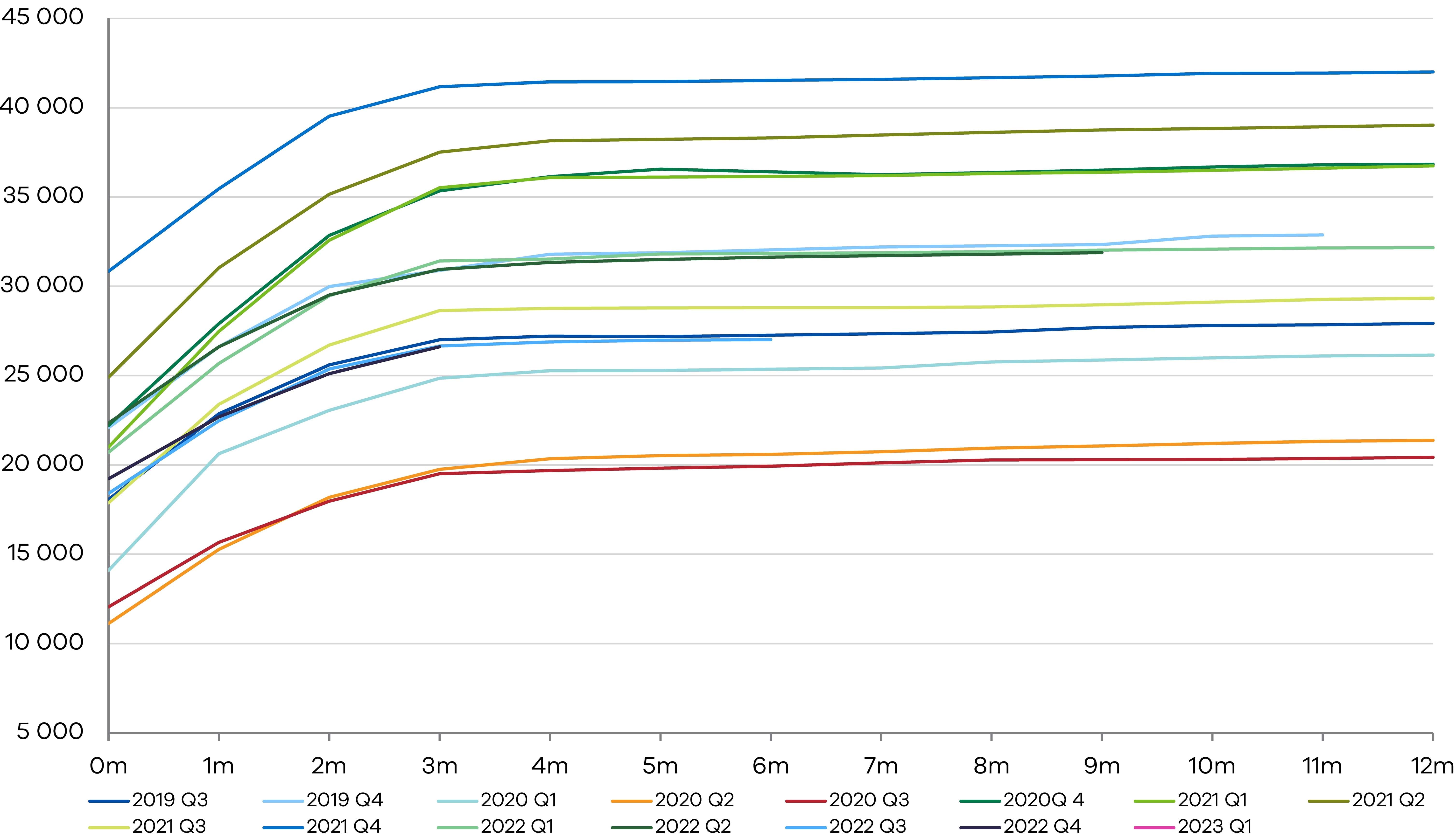
Table 2: Average share of transactions that are received relative to final vintage
Months ahead | Share of total volume in data release 12 months later | Months ahead | Share of total volume in data release 12 months later |
0m | 62% | 6m | 97% |
1m | 79% | 7m | 98% |
2m | 90% | 8m | 99% |
3m | 95% | 9m | 99% |
4m | 97% | 10m | 100% |
5m | 98% | 11m, 12m | 100% |
To estimate the impact of the settlement lag on a hedonic price index, we use the DTF hedonic price index described in Tan and Nasiri (2018). This HPI controls for a variety of factors such as location by postcode, number of bedrooms, bathrooms, car spaces, land size, floor area and age of structure. Using a fixed effects regression, we measure the impacts of each data release through the changes observed in the hedonic price index.
This DTF HPI is estimated for every vintage (i.e. from 0 months ahead, 1 month ahead, and so on to 12 months ahead) to simulate the HPI based on the available information set at each point in time. From here, the through-the-year growth rates are calculated to understand how they evolve across the data vintages. The difference between the HPI growth rate using early vintages relative to the HPI growth rate using final vintage data is calculated for each reference period. The differences are then averaged across reference periods to quantify the evolution to the final growth rate. For example, the 0 months ahead difference for the 2019 Q3, 2019 Q4, …, 2023 Q1 reference periods are averaged to determine the 0 months ahead average difference from the final vintage HPI growth rate. This is repeated for the 1 month ahead, 2 months ahead vintages and so on to the 11 months ahead data vintage. It should be noted that while vintages are released monthly, the HPI is estimated on a quarterly basis.
4. Results
In this section, the results of the data investigations are described by addressing four key questions.
These focus on which property segments dominate early data vintages and are consequently influential in the inferences relied on from analysis of the early vintages. Segments of the property market by region (inner, metro, outer, regional), by property type (houses or units) and by property value (higher or lower contract prices) are examined. The analysis then considers the impact of these vintages on a key metric often used for decision-making where the timeliness of early vintages is useful, such as the DTF HPI.
Do properties in certain regions make up more of the early data?
Figure 2a summarises the average share of properties that settle in the inner, metro and outer Melbourne regions and regional Victoria across vintages while Figure 2b presents the difference of these shares relative to final vintage. It was found that the inner Melbourne region makes up a smaller share of the early data vintages relative to final data vintages. On average, settled inner Melbourne property transactions make up no more than 10 per cent of total settled properties in Victoria, while Melbourne metro, outer Melbourne and regional Victoria account for between 27 and 35 per cent of total properties settled across the data vintages. Properties closer to the CBD make up a larger share of the early data vintages received. On average, the share of properties changed by just under 2 percentage points downward, in the case of inner Melbourne transactions, and upward, for regional Victoria transactions, between the first vintage and final vintage. Appendix Tables A1–A4 provide more details of these shares across the years.
Figure 2a: Average share of settled properties by regions in Victoria, across data vintages
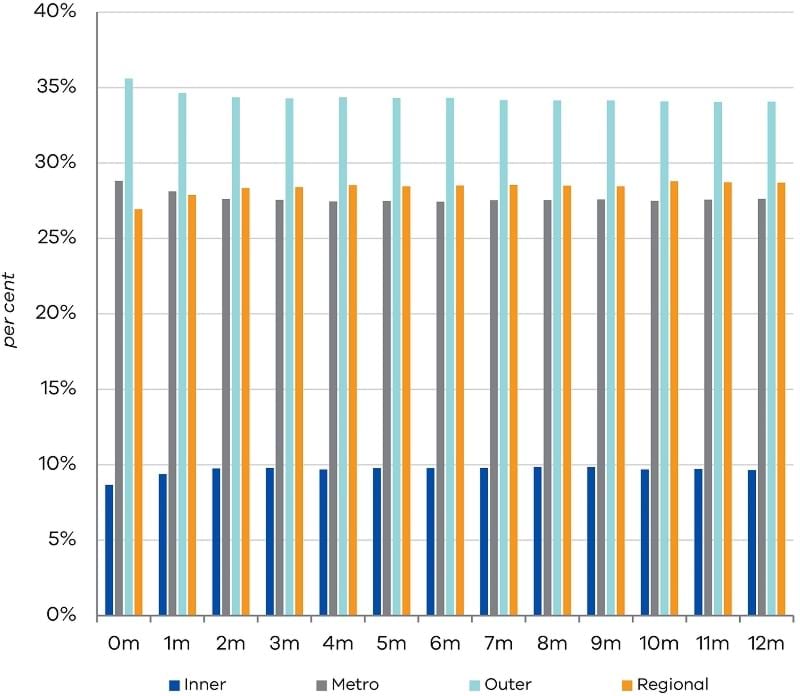
Figure 2b: Average share differences from final vintage
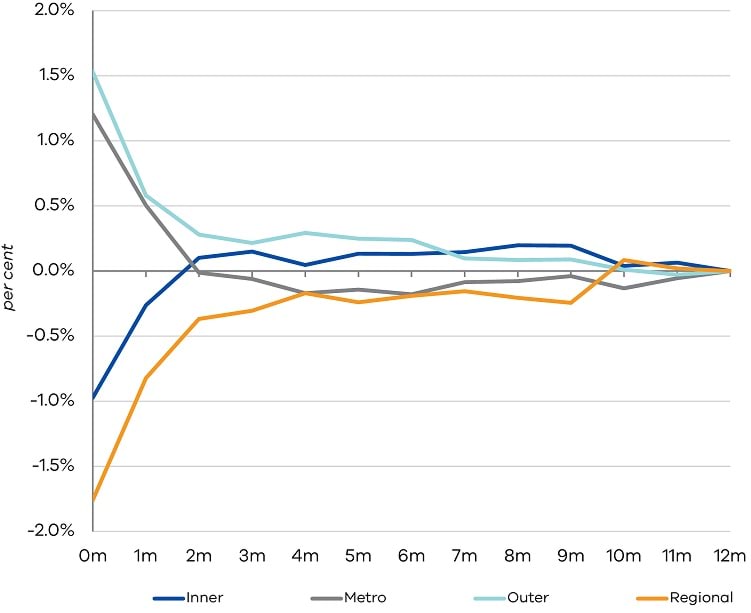
Do houses or units make up more of the early data vintages?
In terms of property types, houses (detached) tend to settle first compared to units (non-detached). As shown in Figure 3, the share of the houses settled is larger in earlier vintages and slowly falls. As we are only considering residential properties that are made up of either houses or units, this suggests that the share of settled unit sales increases over time (and vintages). Appendix Table A5 provides detailed values of these shares over time. On average, the share of houses in the first vintage of settled sales data is approximately 70 per cent. This decreases slightly to about 68 per cent in the final vintage.
Figure 3: Average share of settled properties by type in Victoria, across data vintages
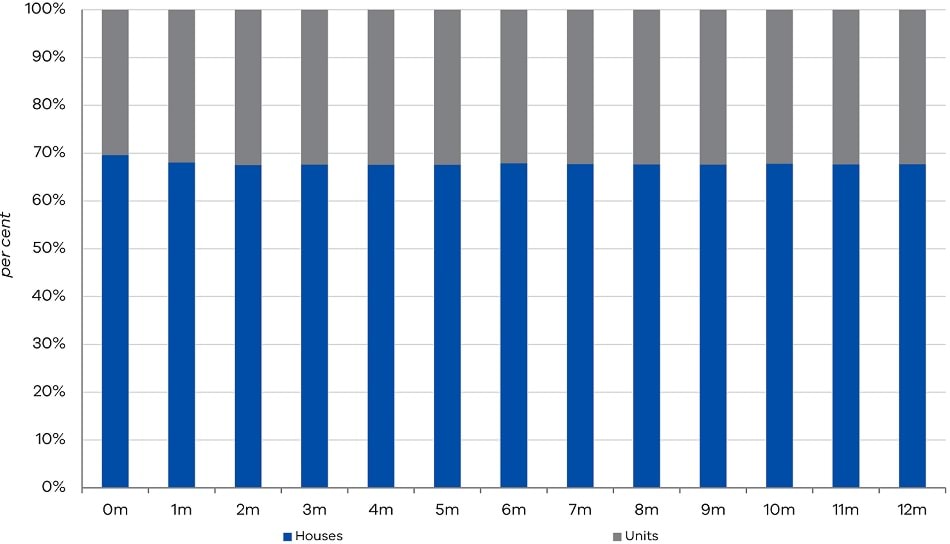
Do higher value properties make up more of the early data vintages?
From Table 3, it appears that higher value properties are settled and recorded first. While there is some variation, as can be seen in the summary table below, the median price across vintages for the same reference period falls by the twelfth month, after the first data release. However, it is possible that this result captures in some part that houses, which are more expensive than units, settle first.
Figure 4 plots the median price of houses and units across vintages to examine this question. Across all the reference quarters, it was clear that the median price of houses transacted decreases with each additional vintage. Thus, more expensive houses settle first, on average. However, the median price of units transacted is equally likely to increase as it is to decrease between first and final vintage. Tables A6 and A7 provide greater detail of the median price changes between the 0 months ahead data vintage and the 12 months ahead data vintage for houses and units, respectively.
Table 3: Median price of settled sales transaction across vintages, by reference quarter
0 months ahead | 12 months ahead | |
2019 Q3 | $580 000 | $570 000 |
2019 Q4 | $615 000 | $601 000 |
2020 Q1 | $610 000 | $590 000 |
2020 Q2 | $597 500 | $590 000 |
2020 Q3 | $562 500 | $552 500 |
2020 Q4 | $629 950 | $620 000 |
2021 Q1 | $660 000 | $643 000 |
2021 Q2 | $685 000 | $663 000 |
2021 Q3 | $672 000 | $660 000 |
2021 Q4 | $730 000 | $715 000 |
2022 Q1 | $720 000 | $700 501 |
2022 Q2 | $720 000 | |
2022 Q3 | $685 000 | |
2022 Q4 | $695 500 | |
2023 Q1 | $680 000 |
Figure 4: Median price differences between first and final data vintage, by property type
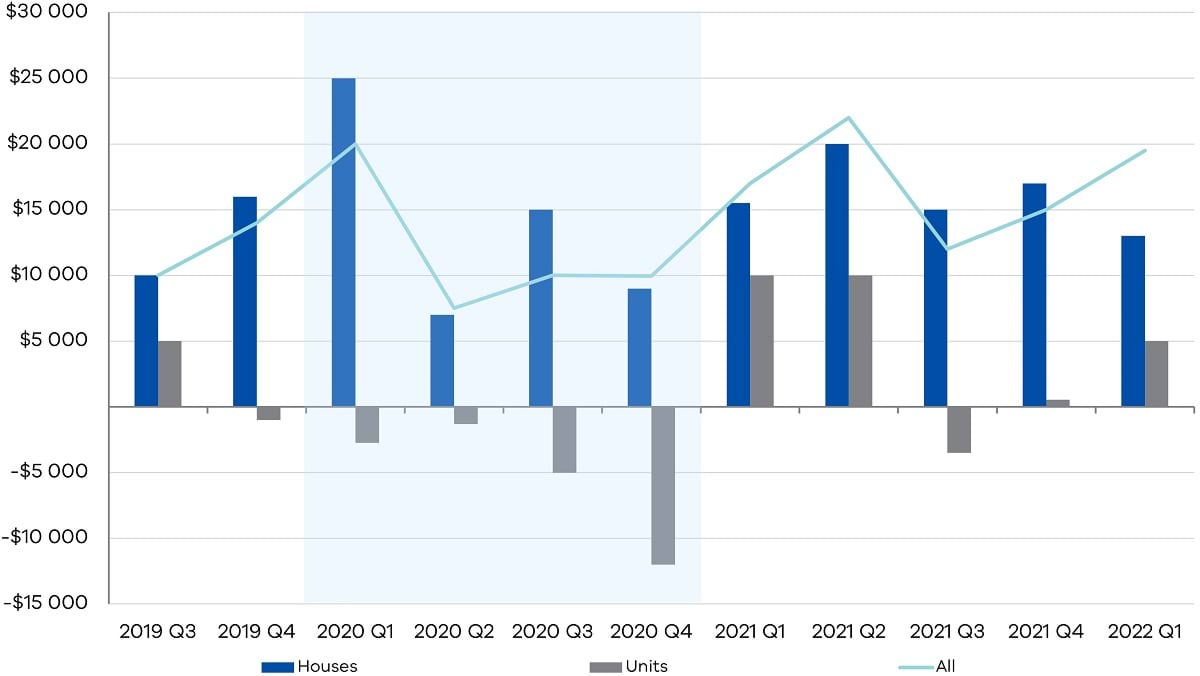
How does the settlement lag impact on the HPI growth rate?
Based on the estimated HPI growth rates, the average size of the difference from final vintage (assumed to be the vintage at least 12 months from first vintage) is calculated and evaluated. We expect that the earlier estimates would be different to later estimates, since there is incomplete data being used in the early stages. We further expect that the size of these differences will shrink as the vintages used to determine the values approach final vintage. Figure 5 summarises the through‑the‑year growth rate differences for each reference quarter across vintages relative to the final through-the-year growth rate. For most of these reference quarters, the estimated growth rate increases as more settled sales data is received.
Figure 5: Evolution of through-the-year growth estimate difference from the final estimate across vintages, by reference quarter
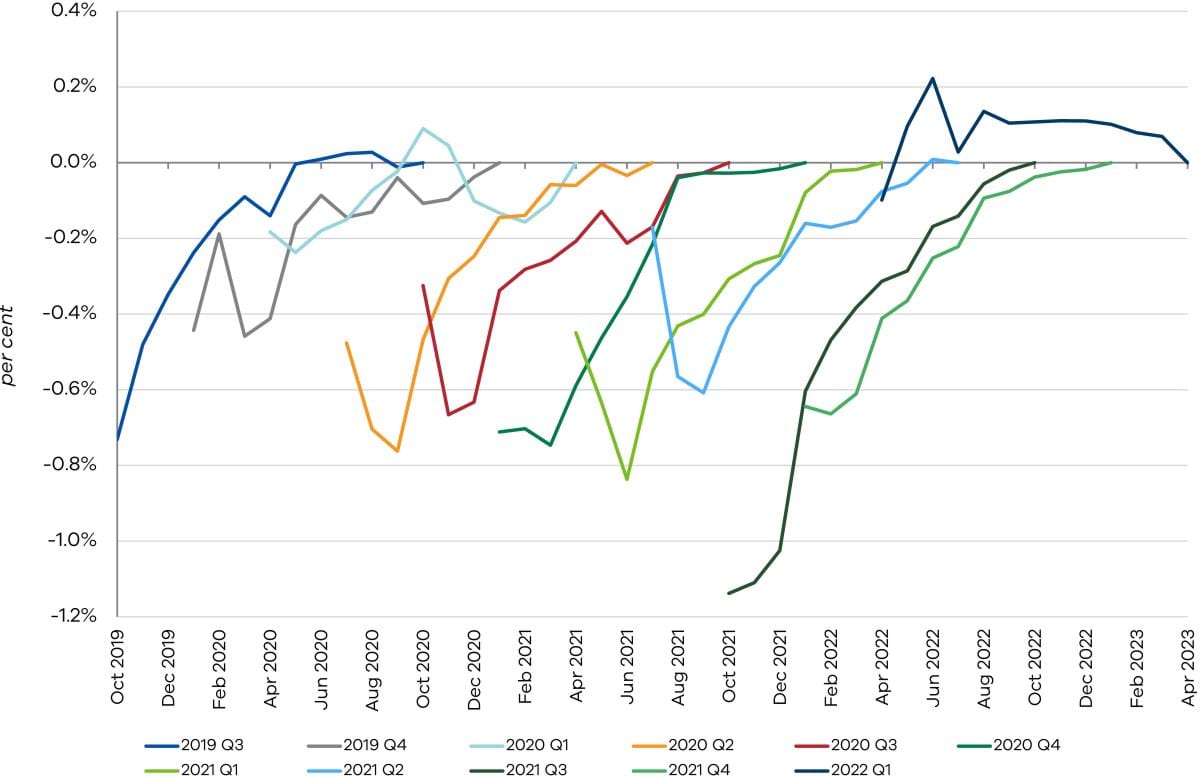
Figure 6 charts the average differences between the HPI growth rate values (based on the reference period’s first 11 vintages) and the corresponding HPI values (based on final data vintage). We can see that the average size of these differences ranges from 0.03 percentage points to 0.62 percentage points. This means that over the period analysed, the HPI growth rate was revised upward as a result of the additional settled sales data received. The biggest difference in the calculated early vintage and final vintage HPI values is surprisingly not found in the first (0 months ahead) or second (1 month ahead) vintages, but rather is in the third vintage (2 months ahead). But as expected, the size of this difference falls to less than 0.1 percentage points by the ninth vintage (8 months ahead).
Figure 6: Average size of difference from final vintage estimate of HPI through-the-year growth
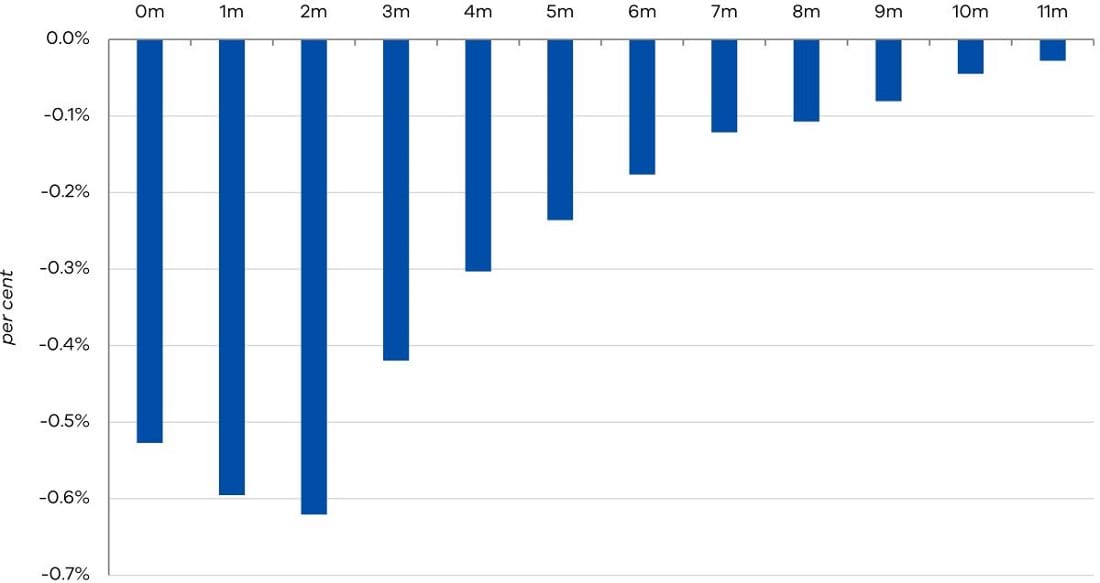
Figure 7 breaks down these HPI estimate differences by region and dwelling type, with some interesting results. We find that early estimates of price growth for inner Melbourne houses require the largest upward revisions compared to other property types. The required growth rate adjustment can be as much as 3 percentage points to match the final vintage values. This large gap appears to persist well into the 7th vintage (6 months ahead). Metro and outer Melbourne houses also need some upward adjustment, but only by up to 0.9 percentage points. This difference is seen to dissipate quickly over the early data vintages.
Figure 7: Average size of difference of estimate of HPI through-the-year growth rate from final estimate
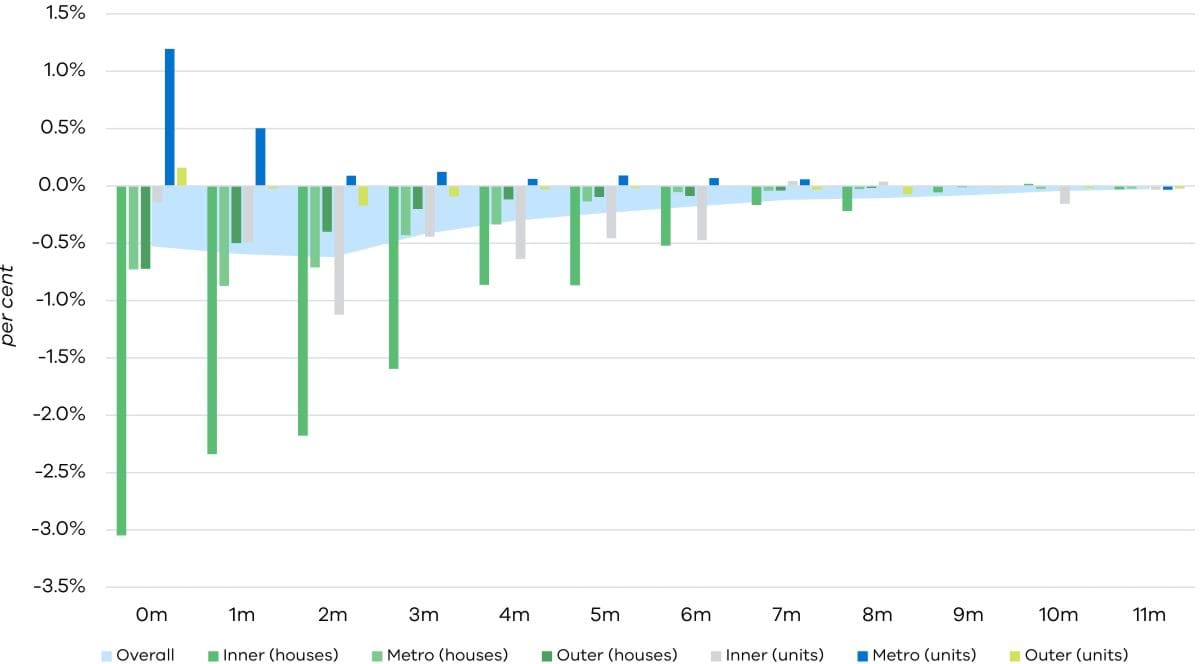
Price growth estimates for units show a different trend altogether, particularly for metro Melbourne units. We find that early vintage data for units or apartments in metro Melbourne indicate a house price growth that is 1.2 percentage points higher than the price estimates gathered from the final vintage data. However, this difference drops quickly to around 0.5 percentage points difference by the second vintage (1 month ahead) and disappears altogether by the fifth vintage (4 months ahead). Appendix Table A8 and A9 provide more growth rate details across the reference quarters.
The average size of the difference is less than 1 full percentage point in growth. The only differences larger than this were found in the inner and metro regions, for both houses and units. This suggests that while only about 60 per cent of final vintage settled sales data is received in the first vintage on average, this partial dataset contains sufficient information to be representative of the final vintage data and inferences made when analysing the overall index.
5. Concluding remarks
In this paper, we investigated settlement lag and the impacts it has on the settled sales data used as the basis for property market forecasts and decision-making.
To our knowledge, analysis on this topic has been very limited. Most certainly, our study is the first of its kind using Australian data.
Over the period that was analysed, it was found that inner Melbourne property transactions make up no more than 10 per cent of total settled properties in the state, while metro Melbourne, outer Melbourne and regional Victoria account for 28, 34 and 29 per cent of all settled sales. Across vintages, properties in inner Melbourne make up a smaller share of the early data vintages relative to the numbers reported in the final data vintages. Our data analysis also found that higher value houses make up a larger share of the early data vintages. However, the share of properties settling by location and type appears to stay the same.
On the impact of settlement lag on HPI values, our analysis shows that early estimates of price growth for inner Melbourne houses require the largest upward revisions, compared to other property types. The required growth rate adjustment can be as much as 3 percentage points, which is four times the rate difference found for other property types. This large gap appears to persist well into the seventh vintage (6 months ahead). Metro and outer Melbourne houses also need some upward adjustment, but only by some 0.9 percentage points, at most. This difference also dissipates more quickly.
Price growth estimates for units show a different trend altogether, particularly for metro Melbourne units. We find that early vintage data for units or apartments in metro Melbourne indicate a price growth that is 1.2 percentage points higher than price growth estimates using final vintage data. This falls quickly though, to just around a 0.5 percentage points difference by the second vintage (1 month ahead) and disappears altogether by the fifth vintage (4 months ahead).
Our findings strongly confirm the validity of our data approach to HPI projections. While the first vintage only contains about 60 per cent of total property transactions on average, our results indicate that it contains sufficient information for early estimates, such as for DTF’s HPI through-the-year growth rate, to not change significantly. The average size of revisions relative to final vintage estimates are less than 1 percentage point.
While this is a reassuring result, it could be worthwhile revisiting this analysis at a future date, with additional vintages and under different property market and economic conditions. This analysis covers only a short period of time, with data vintages captured during a pandemic that was disruptive for the property market.
6. References
References for this Victoria’s Economic Bulletin research article.
Alonso W (1964) ‘Location and Land Use: Toward a General Theory of Land Rent’, Harvard University Press, Cambridge.
Anenberg and Laufer (2017) ‘A More Timely Price Index’, The Review of Economics and Statistics, October 2017, 99(4): 722-734.
Asabere P K and Huffman, F E (1993) ‘The impact of settlement period on sales price’. The Journal of Real Estate Finance and Economics, 7(3), 213-219.
Canstar (2022) ‘Property settlement: What is it and how does it work?(opens in a new window)’ Canstar, accessed 19 February 2022.
Croushore D (2011) ‘Frontiers of real-time data analysis’, Journal of economic literature, 49(1): 72‑100.
Mills E S (1967) ‘An Aggregative Model of Resource Allocation in a Metropolitan Area’, The American Economic Review, Papers and Proceedings, 57(2): 197–210.
Muth R F (1969) ‘Cities and Housing: The Spatial Pattern of Urban Residential Land Use, Third Series: Studies in Business and Society’, University of Chicago Press, Chicago.
National Australia Bank (NAB) (2022) ‘What to know for a hassle-free house settlement’(opens in a new window) NAB, accessed 19 February 2022.
PEXA Insights and Domain (2021) ‘Closing the loop on the property journey’(opens in a new window), accessed April 2022.
Reserve Bank of Australia (RBA) (2004). ‘Measuring Housing Prices’, RBA Bulletin - July 2004, accessed March 2022
Shoory M (2016) ‘The Growth in Apartment Construction in Australia’, RBA Bulletin – June 2016, pages 19–26.
Tan M and Nasiri M (2018) ‘Follow the leader: Exploring leading indicators of the Victorian property market’, Victoria’s Economic Bulletin, 2, 2-21.
Wheaton W C (1974). ‘A Comparative Static Analysis of Urban Spatial Structure’, Journal of Economic Theory, 9(2): 223–237.
7. Appendix
Appendix for this Victoria’s Economic Bulletin research article.
Table A1: Inner Melbourne: share of properties that settle across vintages (per cent)
0m | 1m | 2m | 3m | 4m | 5m | 6m | 7m | 8m | 9m | 10m | 11m | 12m | |
2019 Q3 | 8.62 | 9.27 | 9.44 | 9.69 | 9.79 | 9.85 | 9.36 | 9.98 | 9.96 | 10.06 | 10.15 | 10.21 | 10.21 |
2019 Q4 | 9.02 | 9.67 | 10.11 | 9.40 | 10.22 | 10.22 | 10.37 | 10.49 | 10.57 | 10.57 | 11.35 | 11.39 | 10.50 |
2020 Q1 | 8.78 | 10.01 | 9.77 | 10.22 | 10.15 | 10.06 | 10.05 | 10.09 | 10.14 | 9.84 | 9.86 | 9.96 | 10.04 |
2020 Q2 | 9.02 | 9.05 | 9.13 | 9.20 | 9.19 | 9.57 | 9.41 | 9.61 | 9.81 | 9.83 | 9.97 | 10.04 | 10.16 |
2020 Q3 | 6.44 | 6.80 | 6.72 | 6.55 | 6.53 | 6.64 | 6.70 | 6.74 | 6.97 | 7.02 | 7.06 | 7.09 | 7.08 |
2020 Q4 | 8.00 | 8.09 | 8.68 | 8.86 | 8.86 | 9.09 | 9.16 | 9.27 | 9.23 | 9.25 | 9.22 | 9.21 | 9.19 |
2021 Q1 | 7.67 | 8.82 | 9.23 | 9.17 | 9.26 | 9.32 | 9.33 | 9.31 | 9.30 | 9.31 | 9.32 | 9.31 | 9.32 |
2021 Q2 | 8.49 | 9.98 | 10.33 | 10.26 | 10.19 | 10.22 | 10.26 | 10.26 | 10.24 | 10.30 | 10.28 | 10.27 | 10.27 |
2021 Q3 | 7.61 | 9.00 | 9.27 | 9.19 | 9.19 | 9.21 | 9.19 | 9.18 | 9.25 | 9.22 | 8.90 | 8.86 | 8.85 |
2021 Q4 | 8.28 | 9.18 | 9.60 | 9.74 | 9.75 | 9.77 | 9.79 | 9.78 | 9.77 | 9.76 | 9.77 | 9.82 | 9.81 |
2022 Q1 | 8.86 | 10.41 | 11.42 | 11.48 | 10.63 | 10.54 | 10.50 | 10.51 | 10.57 | 10.58 | 10.59 | 10.59 | 10.62 |
2022 Q2 | 10.68 | 10.69 | 11.04 | 11.28 | 11.52 | 11.86 | 12.14 | 12.19 | 12.25 | 12.28 | |||
2022 Q3 | 8.71 | 9.80 | 10.37 | 10.47 | 10.66 | 10.72 | 10.76 | ||||||
2022 Q4 | 9.58 | 10.53 | 11.28 | 11.54 | |||||||||
2023 Q1 | 10.23 | ||||||||||||
Average share | 8.67 | 9.38 | 9.74 | 9.79 | 9.69 | 9.77 | 9.77 | 9.79 | 9.84 | 9.84 | 9.68 | 9.70 | 9.64 |
Table A2: Metro: share of properties that settle across vintages (per cent)
0m | 1m | 2m | 3m | 4m | 5m | 6m | 7m | 8m | 9m | 10m | 11m | 12m | |
2019 Q3 | 30.93 | 29.19 | 28.84 | 29.11 | 29.23 | 29.07 | 28.45 | 29.10 | 29.12 | 29.00 | 29.05 | 29.06 | 29.20 |
2019 Q4 | 32.11 | 31.34 | 30.69 | 30.46 | 30.86 | 30.83 | 30.69 | 30.75 | 30.76 | 30.84 | 30.54 | 30.55 | 30.91 |
2020 Q1 | 28.20 | 27.60 | 27.04 | 27.09 | 27.14 | 26.98 | 26.91 | 26.91 | 26.93 | 27.22 | 27.34 | 27.37 | 27.19 |
2020 Q2 | 30.18 | 28.96 | 28.37 | 28.23 | 27.84 | 27.77 | 27.93 | 27.95 | 27.85 | 27.77 | 27.71 | 27.84 | 27.81 |
2020 Q3 | 22.68 | 21.69 | 20.77 | 20.65 | 20.64 | 20.60 | 20.48 | 20.48 | 20.53 | 20.60 | 20.70 | 20.81 | 20.89 |
2020 Q4 | 27.28 | 26.55 | 25.96 | 25.52 | 25.53 | 25.54 | 25.61 | 25.90 | 26.00 | 26.03 | 26.16 | 26.16 | 26.19 |
2021 Q1 | 28.25 | 27.05 | 26.32 | 26.38 | 26.55 | 26.61 | 26.60 | 26.61 | 26.64 | 26.69 | 26.71 | 26.71 | 26.78 |
2021 Q2 | 30.29 | 29.81 | 29.20 | 29.11 | 29.13 | 29.10 | 29.19 | 29.25 | 29.25 | 29.31 | 29.35 | 29.36 | 29.39 |
2021 Q3 | 27.25 | 26.70 | 26.33 | 26.29 | 26.22 | 26.27 | 26.32 | 26.34 | 26.39 | 26.43 | 26.53 | 27.18 | 27.19 |
2021 Q4 | 31.55 | 30.89 | 30.47 | 30.43 | 30.40 | 30.41 | 30.43 | 30.62 | 30.65 | 30.69 | 30.78 | 30.65 | 30.67 |
2022 Q1 | 27.69 | 27.21 | 26.68 | 26.60 | 26.88 | 27.50 | 27.45 | 27.46 | 27.41 | 27.37 | 27.38 | 27.40 | 27.48 |
2022 Q2 | 29.75 | 29.40 | 29.11 | 28.98 | 29.05 | 28.95 | 28.92 | 28.90 | 28.87 | 28.89 | |||
2022 Q3 | 28.00 | 27.62 | 27.11 | 27.33 | 27.26 | 27.44 | 27.64 | ||||||
2022 Q4 | 30.60 | 29.60 | 29.45 | 29.52 | |||||||||
2023 Q1 | 27.40 | ||||||||||||
Average share | 28.81 | 28.11 | 27.60 | 27.55 | 27.44 | 27.47 | 27.43 | 27.52 | 27.53 | 27.57 | 27.48 | 27.55 | 27.61 |
Table A3: Outer: share of properties that settle across vintages (per cent)
0m | 1m | 2m | 3m | 4m | 5m | 6m | 7m | 8m | 9m | 10m | 11m | 12m | |
2019 Q3 | 34.46 | 34.38 | 34.49 | 34.17 | 34.11 | 34.16 | 34.11 | 34.19 | 34.20 | 34.29 | 34.23 | 34.20 | 34.11 |
2019 Q4 | 33.65 | 32.93 | 32.88 | 32.84 | 32.83 | 32.77 | 32.85 | 32.79 | 32.75 | 32.71 | 32.49 | 32.46 | 32.75 |
2020 Q1 | 34.11 | 33.59 | 33.92 | 33.85 | 33.89 | 34.10 | 34.11 | 34.17 | 34.11 | 34.11 | 34.08 | 34.03 | 34.13 |
2020 Q2 | 34.04 | 34.15 | 34.08 | 34.39 | 34.81 | 34.59 | 34.59 | 34.54 | 34.57 | 34.71 | 34.79 | 34.69 | 34.68 |
2020 Q3 | 31.78 | 30.05 | 29.61 | 29.08 | 28.94 | 29.07 | 29.35 | 29.58 | 29.53 | 29.46 | 29.30 | 29.27 | 29.33 |
2020 Q4 | 35.17 | 35.19 | 34.70 | 34.95 | 34.96 | 34.78 | 34.51 | 34.03 | 33.98 | 34.00 | 34.01 | 34.01 | 34.05 |
2021 Q1 | 36.53 | 35.11 | 34.65 | 34.87 | 34.71 | 34.69 | 34.73 | 34.76 | 34.76 | 34.74 | 34.74 | 34.81 | 34.83 |
2021 Q2 | 36.58 | 34.45 | 34.12 | 34.09 | 34.05 | 34.12 | 34.06 | 34.04 | 34.12 | 34.08 | 34.10 | 34.09 | 34.09 |
2021 Q3 | 37.13 | 35.96 | 35.61 | 35.55 | 35.69 | 35.71 | 35.74 | 35.79 | 35.68 | 35.71 | 35.87 | 35.54 | 35.57 |
2021 Q4 | 36.56 | 35.99 | 35.47 | 35.24 | 35.32 | 35.29 | 35.31 | 35.24 | 35.24 | 35.24 | 35.22 | 35.29 | 35.30 |
2022 Q1 | 37.94 | 36.61 | 35.82 | 35.82 | 36.12 | 35.83 | 35.88 | 35.92 | 35.95 | 35.95 | 35.94 | 35.96 | 35.81 |
2022 Q2 | 35.77 | 35.41 | 35.23 | 35.19 | 35.06 | 34.93 | 34.84 | 34.82 | 34.83 | 34.77 | |||
2022 Q3 | 37.74 | 36.66 | 36.30 | 36.14 | 36.09 | 35.97 | 35.78 | ||||||
2022 Q4 | 35.06 | 34.48 | 33.87 | 33.68 | |||||||||
2023 Q1 | 37.34 | ||||||||||||
Average share | 35.59 | 34.64 | 34.34 | 34.27 | 34.35 | 34.31 | 34.30 | 34.16 | 34.14 | 34.15 | 34.07 | 34.03 | 34.06 |
Table A4: Regional: share of properties that settle across vintages (per cent)
0m | 1m | 2m | 3m | 4m | 5m | 6m | 7m | 8m | 9m | 10m | 11m | 12m | |
2019 Q3 | 25.99 | 27.16 | 27.22 | 27.02 | 26.88 | 26.92 | 28.07 | 26.73 | 26.71 | 26.65 | 26.57 | 26.53 | 26.49 |
2019 Q4 | 25.21 | 26.06 | 26.33 | 27.30 | 26.08 | 26.19 | 26.09 | 25.97 | 25.92 | 25.89 | 25.61 | 25.61 | 25.84 |
2020 Q1 | 28.91 | 28.81 | 29.27 | 28.85 | 28.83 | 28.86 | 28.94 | 28.82 | 28.83 | 28.83 | 28.72 | 28.64 | 28.63 |
2020 Q2 | 26.76 | 27.85 | 28.42 | 28.17 | 28.15 | 28.07 | 28.06 | 27.90 | 27.76 | 27.69 | 27.54 | 27.43 | 27.35 |
2020 Q3 | 39.10 | 41.47 | 42.90 | 43.73 | 43.89 | 43.69 | 43.47 | 43.20 | 42.97 | 42.92 | 42.94 | 42.83 | 42.70 |
2020 Q4 | 29.55 | 30.17 | 30.66 | 30.67 | 30.65 | 30.59 | 30.71 | 30.79 | 30.78 | 30.71 | 30.61 | 30.62 | 30.58 |
2021 Q1 | 27.55 | 29.03 | 29.81 | 29.58 | 29.47 | 29.38 | 29.34 | 29.31 | 29.30 | 29.26 | 29.23 | 29.18 | 29.07 |
2021 Q2 | 24.63 | 25.76 | 26.35 | 26.55 | 26.63 | 26.55 | 26.49 | 26.45 | 26.39 | 26.31 | 26.27 | 26.28 | 26.25 |
2021 Q3 | 28.01 | 28.35 | 28.80 | 28.97 | 28.90 | 28.81 | 28.76 | 28.69 | 28.69 | 28.64 | 28.70 | 28.43 | 28.38 |
2021 Q4 | 23.60 | 23.94 | 24.46 | 24.58 | 24.53 | 24.52 | 24.48 | 24.36 | 24.34 | 24.31 | 24.24 | 24.24 | 24.22 |
2022 Q1 | 25.51 | 25.77 | 26.08 | 26.10 | 26.37 | 26.14 | 26.16 | 26.10 | 26.08 | 26.10 | 26.09 | 26.05 | 26.09 |
2022 Q2 | 23.80 | 24.50 | 24.62 | 24.56 | 24.38 | 24.26 | 24.11 | 24.09 | 24.06 | 24.06 | |||
2022 Q3 | 25.54 | 25.92 | 26.22 | 26.06 | 26.00 | 25.88 | 25.83 | ||||||
2022 Q4 | 24.76 | 25.38 | 25.40 | 25.26 | |||||||||
2023 Q1 | 25.03 | ||||||||||||
Average share | 26.93 | 27.87 | 28.32 | 28.39 | 28.52 | 28.45 | 28.50 | 28.54 | 28.49 | 28.45 | 28.77 | 28.71 | 28.69 |
Table A5: Share of houses settled in each vintage (per cent)
0m | 1m | 2m | 3m | 4m | 5m | 6m | 7m | 8m | 9m | 10m | 11m | 12m | |
2019 Q3 | 69.34 | 68.21 | 67.62 | 67.21 | 66.95 | 67.06 | 70.31 | 66.67 | 66.59 | 66.42 | 66.24 | 66.24 | 66.14 |
2019 Q4 | 69.51 | 68.16 | 67.80 | 70.88 | 66.91 | 66.74 | 66.74 | 66.64 | 66.58 | 66.53 | 65.94 | 65.86 | 66.47 |
2020 Q1 | 72.77 | 67.74 | 67.63 | 67.00 | 66.99 | 67.12 | 67.18 | 67.18 | 67.09 | 67.18 | 67.03 | 66.91 | 66.94 |
2020 Q2 | 69.04 | 68.91 | 68.62 | 68.48 | 68.92 | 68.79 | 68.99 | 68.72 | 68.54 | 68.62 | 68.51 | 68.39 | 68.31 |
2020 Q3 | 72.23 | 72.01 | 72.48 | 72.76 | 72.88 | 72.96 | 73.14 | 73.10 | 73.01 | 73.12 | 72.86 | 72.78 | 72.68 |
2020 Q4 | 71.31 | 70.86 | 70.43 | 70.72 | 70.94 | 70.74 | 70.83 | 70.43 | 70.39 | 70.36 | 70.37 | 70.30 | 70.34 |
2021 Q1 | 71.21 | 68.90 | 68.11 | 68.20 | 67.94 | 68.05 | 68.20 | 68.23 | 68.24 | 68.22 | 68.10 | 68.03 | 67.96 |
2021 Q2 | 69.36 | 66.43 | 66.03 | 66.02 | 66.28 | 66.41 | 66.48 | 66.38 | 66.33 | 66.23 | 66.22 | 66.19 | 66.16 |
2021 Q3 | 69.72 | 67.21 | 66.93 | 67.11 | 67.15 | 67.29 | 67.26 | 67.30 | 67.26 | 67.16 | 67.41 | 66.86 | 66.80 |
2021 Q4 | 69.66 | 68.01 | 67.16 | 66.77 | 66.87 | 66.86 | 66.96 | 66.78 | 66.77 | 66.70 | 66.66 | 66.61 | 66.59 |
2022 Q1 | 69.08 | 66.56 | 65.30 | 65.01 | 65.78 | 66.12 | 66.07 | 66.01 | 66.03 | 65.99 | 65.97 | 65.94 | 66.07 |
2022 Q2 | 67.11 | 65.93 | 65.45 | 65.38 | 65.09 | 64.92 | 64.80 | 64.77 | 64.69 | 64.64 | |||
2022 Q3 | 68.68 | 66.66 | 65.75 | 65.51 | 65.51 | 65.23 | 65.25 | ||||||
2022 Q4 | 68.07 | 66.83 | 65.57 | 65.12 | |||||||||
2023 Q1 | 66.80 | ||||||||||||
Average share | 69.59 | 68.03 | 67.49 | 67.58 | 67.55 | 67.56 | 67.86 | 67.69 | 67.63 | 67.60 | 67.76 | 67.65 | 67.68 |
Table A6: Houses: Median price of settled sales transaction across vintages, by reference quarter
0 months ahead | 12 months ahead | |
2019 Q3 | $600 000 | $590 000 |
2019 Q4 | $641 000 | $625 000 |
2020 Q1 | $635 000 | $610 000 |
2020 Q2 | $612 000 | $605 000 |
2020 Q3 | $580 000 | $565 000 |
2020 Q4 | $655 000 | $646 000 |
2021 Q1 | $695 000 | $679 500 |
2021 Q2 | $730 000 | $710 000 |
2021 Q3 | $715 000 | $700 000 |
2021 Q4 | $795 000 | $778 000 |
2022 Q1 | $778 000 | $765 000 |
2022 Q2 | $790 000 | |
2022 Q3 | $740 000 | |
2022 Q4 | $752 000 | |
2023 Q1 | $740 000 |
Table A7: Units – Median price of settled sales transaction across vintages, by reference quarter
0 months ahead | 12 months ahead | |
2019 Q3 | $530 000 | $525 000 |
2019 Q4 | $560 000 | $561 000 |
2020 Q1 | $547 250 | $550 000 |
2020 Q2 | $550 000 | $551 300 |
2020 Q3 | $520 000 | $525 000 |
2020 Q4 | $558 000 | $570 000 |
2021 Q1 | $590 000 | $580 000 |
2021 Q2 | $600 000 | $590 000 |
2021 Q3 | $576 500 | $580 000 |
2021 Q4 | $615 550 | $615 000 |
2022 Q1 | $600 000 | $595 000 |
2022 Q2 | $596 000 | |
2022 Q3 | $570 000 | |
2022 Q4 | $575 000 | |
2023 Q1 | $560 000 |
Table A8: Average size of difference from final vintage estimate of HPI through-the-year growth (per cent)
0m | 1m | 2m | 3m | 4m | 5m | 6m | 7m | 8m | 9m | 10m | 11m | |
2019 Q3 | -0.73 | -0.48 | -0.35 | -0.24 | -0.15 | -0.09 | -0.14 | 0.00 | 0.01 | 0.02 | 0.03 | -0.01 |
2019 Q4 | -0.44 | -0.19 | -0.46 | -0.41 | -0.16 | -0.09 | -0.14 | -0.13 | -0.04 | -0.11 | -0.10 | -0.04 |
2020 Q1 | -0.18 | -0.24 | -0.18 | -0.15 | -0.07 | -0.02 | 0.09 | 0.05 | -0.10 | -0.13 | -0.16 | -0.11 |
2020 Q2 | -0.48 | -0.70 | -0.76 | -0.47 | -0.31 | -0.25 | -0.14 | -0.14 | -0.06 | -0.06 | 0.00 | -0.03 |
2020 Q3 | -0.32 | -0.67 | -0.63 | -0.34 | -0.28 | -0.26 | -0.21 | -0.13 | -0.21 | -0.17 | -0.04 | -0.03 |
2020 Q4 | -0.71 | -0.70 | -0.75 | -0.59 | -0.46 | -0.35 | -0.22 | -0.04 | -0.03 | -0.03 | -0.03 | -0.02 |
2021 Q1 | -0.45 | -0.63 | -0.84 | -0.55 | -0.43 | -0.40 | -0.31 | -0.27 | -0.25 | -0.08 | -0.02 | -0.02 |
2021 Q2 | -0.17 | -0.57 | -0.61 | -0.43 | -0.33 | -0.26 | -0.16 | -0.17 | -0.15 | -0.08 | -0.05 | 0.01 |
2021 Q3 | -1.14 | -1.11 | -1.03 | -0.60 | -0.47 | -0.38 | -0.31 | -0.29 | -0.17 | -0.14 | -0.06 | -0.02 |
2021 Q4 | -0.64 | -0.66 | -0.61 | -0.41 | -0.37 | -0.25 | -0.22 | -0.09 | -0.08 | -0.04 | -0.02 | -0.02 |
2022 Q1 | -0.10 | -0.10 | -0.22 | -0.03 | -0.14 | -0.10 | -0.11 | -0.11 | -0.11 | -0.10 | -0.08 | -0.07 |
Average share | -0.48 | -0.70 | -0.76 | -0.47 | -0.31 | -0.25 | -0.14 | -0.14 | -0.06 | -0.06 | 0.00 | -0.03 |
Note: Final vintage (12 months ahead) data is only available up to 2022 Q1.
Table A9: Average size of difference of estimate of HPI through-the-year growth rate from final estimate (per cent)
Months ahead | Overall | Inner (houses) | Metro (houses) | Outer (houses) | Inner (units) | Metro (units) | Outer (units) |
0M | -0.49 | -3.05 | -0.73 | -0.72 | -0.14 | 1.19 | 0.16 |
1M | -0.55 | -2.34 | -0.87 | -0.50 | -0.49 | 0.50 | -0.02 |
2M | -0.58 | -2.18 | -0.71 | -0.40 | -1.12 | 0.09 | -0.17 |
3M | -0.38 | -1.60 | -0.43 | -0.20 | -0.44 | 0.12 | -0.09 |
4M | -0.29 | -0.86 | -0.34 | -0.12 | -0.64 | 0.06 | -0.03 |
5M | -0.22 | -0.87 | -0.13 | -0.10 | -0.46 | 0.09 | -0.02 |
6M | -0.17 | -0.52 | -0.05 | -0.09 | -0.47 | 0.07 | -0.01 |
7M | -0.12 | -0.17 | -0.04 | -0.04 | 0.04 | 0.06 | -0.03 |
8M | -0.11 | -0.22 | -0.03 | -0.02 | 0.04 | 0.00 | -0.07 |
9M | -0.08 | -0.06 | 0.00 | -0.01 | -0.01 | 0.00 | -0.01 |
10M | -0.05 | 0.02 | -0.02 | 0.00 | -0.16 | 0.00 | -0.02 |
11M | -0.03 | -0.03 | -0.02 | 0.00 | -0.03 | -0.03 | -0.02 |