Relying on real-time analysis terminology, each data release is referred to as a ‘vintage’. For example, the first vintage for settled sales contracted in 2019 Q3 will be the October 2019 data release. This is the first monthly data release of settled sales data for the full quarter of 2019 Q3 (up to and including September 2019) that is available. In this example, 2019 Q3 is the ‘reference quarter.’
Based on separate pieces of analysis, almost all transactions are expected to be settled by the twelfth month. A check of the number of transactions at the 12-month mark shows that it is similar to the number of transactions at the 2-year mark. This suggests that the off-the-plan sales that are anticipated to settle only after two or three years (Shoory, 2016) do not make up a significant portion of the market. Therefore, we assume that the final vintage is released at least 12 months after the first vintage is released. For example, the final vintage for the 2019 Q3 reference quarter will be the October 2020 vintage.
To standardise the real-time data for analysis, vintages are described in terms of the number of months ahead relative to the reference quarter plus one month, e.g. the first vintage for the 2019 Q3 reference quarter is the October 2019 data release, which is 0 months ahead. Table 1 summarises the data vintages and number of months ahead in relation to each reference quarter.
In Victoria, there are approximately 10,000 residential transactions on average that settle each month. Figure 1 summarises the volume of transactions that are received over time for each reference quarter while Table 2 displays the share of transactions that are observed relative to final vintage, as an average over the reference quarters. For a given reference quarter, approximately 60 per cent of total settled property transactions present in the final vintage is observed in the first vintage, on average. By the end of the third month, most of the transactions had been received, which is consistent with the average settlement period from Canstar (2022).
Table 1: Data vintages and months ahead by reference quarter
0m | 1m | 2m | 3m | 4m | 5m | 6m | 7m | 8m | 9m | 10m | 11m | 12m | |
2019 Q3 | Oct 19 | Nov 19 | Dec 19 | Jan 20 | Feb 20 | Mar 20 | Apr 20 | May 20 | Jun 20 | Jul 20 | Aug 20 | Sep 20 | Oct 20 |
2019 Q4 | Jan 20 | Feb 20 | Mar 20 | Apr 20 | May 20 | Jun 20 | Jul 20 | Aug 20 | Sep 20 | Oct 20 | Nov 20 | Dec 20 | Jan 21 |
2020 Q1 | Apr 20 | May 20 | Jun 20 | Jul 20 | Aug 20 | Sep 20 | Oct 20 | Nov 20 | Dec 20 | Jan 21 | Feb 21 | Mar 21 | Apr 21 |
2020 Q2 | Jul 20 | Aug 20 | Sep 20 | Oct 20 | Nov 20 | Dec 20 | Jan 21 | Feb 21 | Mar 21 | Apr 21 | May 21 | Jun 21 | Jul 21 |
2020 Q3 | Oct 20 | Nov 20 | Dec 20 | Jan 21 | Feb 21 | Mar 21 | Apr 21 | May 21 | Jun 21 | Jul 21 | Aug 21 | Sep 21 | Oct 21 |
2020 Q4 | Jan 21 | Feb 21 | Mar 21 | Apr 21 | May 21 | Jun 21 | Jul 21 | Aug 21 | Sep 21 | Oct 21 | Nov 21 | Dec 21 | Jan 22 |
2021 Q1 | Apr 21 | May 21 | Jun 21 | Jul 21 | Aug 21 | Sep 21 | Oct 21 | Nov 21 | Dec 21 | Jan 22 | Feb 22 | Mar 22 | Apr 22 |
2021 Q2 | Jul 21 | Aug 21 | Sep 21 | Oct 21 | Nov 21 | Dec 21 | Jan 22 | Feb 22 | Mar 22 | Apr 22 | May 22 | Jun 22 | Jul 22 |
2021 Q3 | Oct 21 | Nov 21 | Dec 21 | Jan 22 | Feb 22 | Mar 22 | Apr 22 | May 22 | Jun 22 | Jul 22 | Aug 22 | Sep 22 | Oct 22 |
2021 Q4 | Jan 22 | Feb 22 | Mar 22 | Apr 22 | May 22 | Jun 22 | Jul 22 | Aug 22 | Sep 22 | Oct 22 | Nov 22 | Dec 22 | Jan 23 |
2022 Q1 | Apr 22 | May 22 | Jun 22 | Jul 22 | Aug 22 | Sep 22 | Oct 22 | Nov 22 | Dec 22 | Jan 23 | Feb 23 | Mar 23 | Apr 23 |
2022 Q2 | Jul 22 | Aug 22 | Sep 22 | Oct 22 | Nov 22 | Dec 22 | Jan 23 | Feb 23 | Mar 23 | Apr 23 | |||
2022 Q3 | Oct 22 | Nov 22 | Dec 22 | Jan 23 | Feb 23 | Mar 23 | Apr 23 | ||||||
2022 Q4 | Jan 23 | Feb 23 | Mar 23 | Apr 23 | |||||||||
2023 Q1 | Apr 23 |
Figure 1: Volume of settled sales transactions across vintages
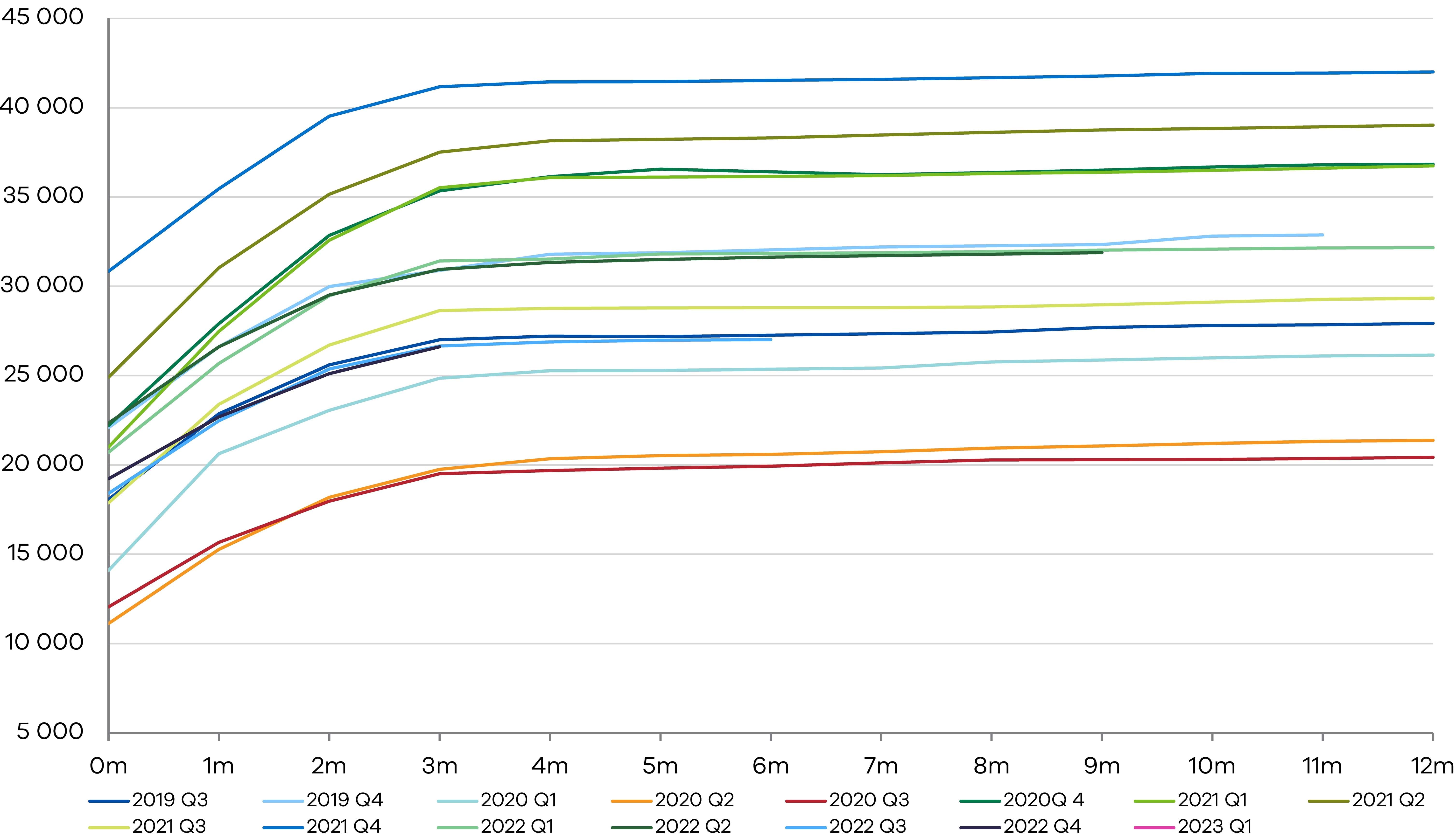
Table 2: Average share of transactions that are received relative to final vintage
Months ahead | Share of total volume in data release 12 months later | Months ahead | Share of total volume in data release 12 months later |
0m | 62% | 6m | 97% |
1m | 79% | 7m | 98% |
2m | 90% | 8m | 99% |
3m | 95% | 9m | 99% |
4m | 97% | 10m | 100% |
5m | 98% | 11m, 12m | 100% |
To estimate the impact of the settlement lag on a hedonic price index, we use the DTF hedonic price index described in Tan and Nasiri (2018). This HPI controls for a variety of factors such as location by postcode, number of bedrooms, bathrooms, car spaces, land size, floor area and age of structure. Using a fixed effects regression, we measure the impacts of each data release through the changes observed in the hedonic price index.
This DTF HPI is estimated for every vintage (i.e. from 0 months ahead, 1 month ahead, and so on to 12 months ahead) to simulate the HPI based on the available information set at each point in time. From here, the through-the-year growth rates are calculated to understand how they evolve across the data vintages. The difference between the HPI growth rate using early vintages relative to the HPI growth rate using final vintage data is calculated for each reference period. The differences are then averaged across reference periods to quantify the evolution to the final growth rate. For example, the 0 months ahead difference for the 2019 Q3, 2019 Q4, …, 2023 Q1 reference periods are averaged to determine the 0 months ahead average difference from the final vintage HPI growth rate. This is repeated for the 1 month ahead, 2 months ahead vintages and so on to the 11 months ahead data vintage. It should be noted that while vintages are released monthly, the HPI is estimated on a quarterly basis.
Updated